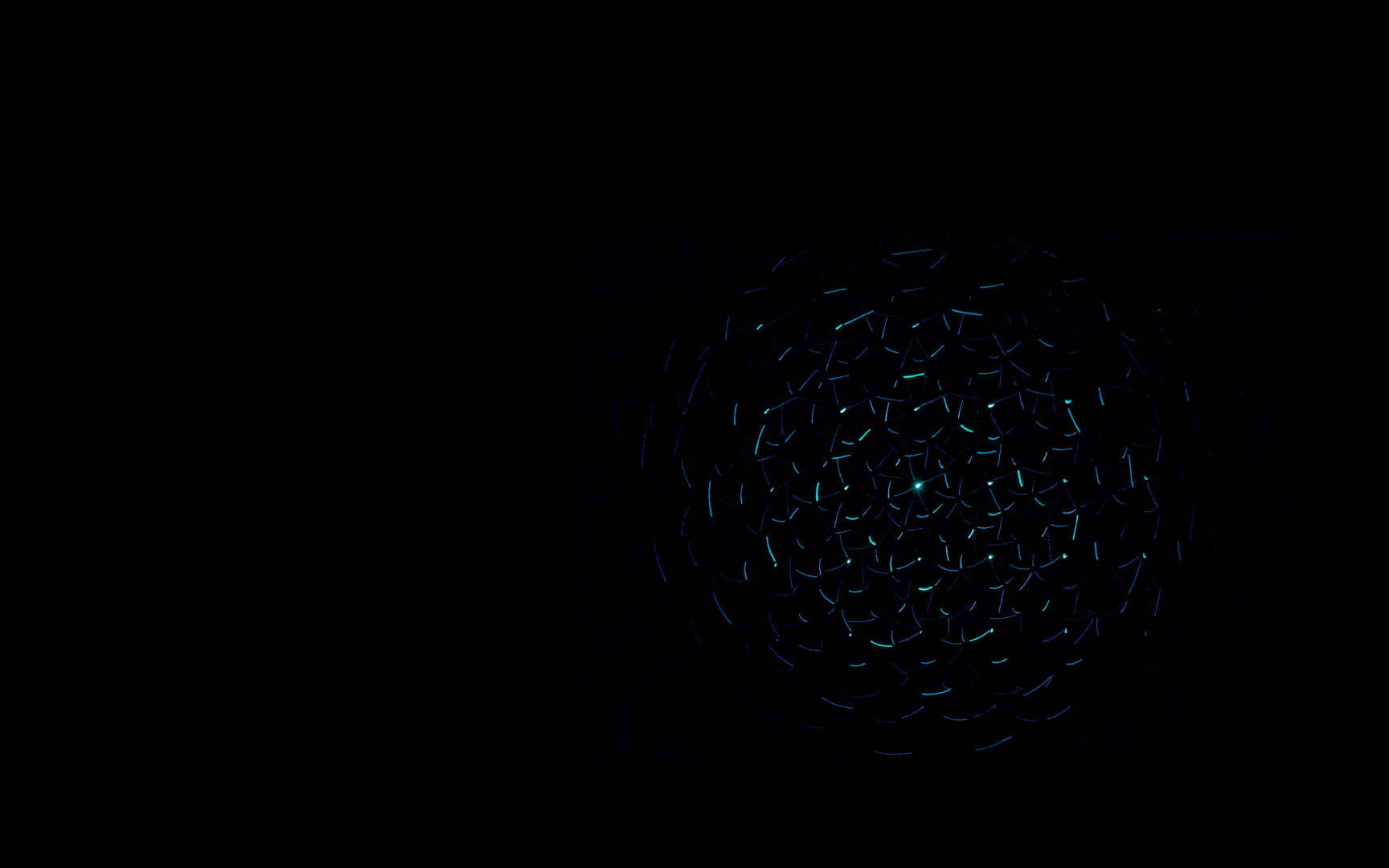
The Rise of Generative AI and its Security Challenges
In this article, we’ll explore Generative AI: what it is, why it’s so popular, the security risks it poses and how we can build a secure future with it.
Generative AI has taken the tech world by storm. By the end of 2023, the global Generative AI market was worth $44.81 billion, nearly doubling from $29 billion in 2022 (Statista). Some predictions suggest it could become a $1.3 trillion market by 2032 (Bloomberg). This rapid growth is largely due to groundbreaking models like ChatGPT, released in 2022, which have revolutionised how many people solve problems and brainstorm ideas. However, despite the surge in interest and investment, a study by Searce showed that 58% of organisations haven’t adopted AI due to cybersecurity concerns.
Understanding Generative AI
Generative Artificial Intelligence describes algorithms that can transform simple prompts into personalised content, drawing from the vast data they’ve been trained on. Where traditional AI excels in handling numerical and optimisation tasks, the value in generative AI is the layer of creativity and innovation it adds. It can generate new images with models like DALL-E, videos with tools like SORA, or human-like text with ChatGPT and Llama. However, this capability comes at a price—training generative AI requires an enormous amount of data, making the process time-intensive, costly, and power-hungry.
Recent Surge in Interest
A study by McKinsey found that 61% of workers surveyed were either using or planning to use generative AI. Some key reasons for this surge in interest are that generative AI can save time, boost productivity, and uncover new opportunities for innovation in the workplace. According to Hubspot, chatbots powered by Generative AI save an average of 2 hours and 11 minutes per day by handling customer queries. Whilst GitHub’s Copilot, an AI coding assistant, has reported making developers 88% more productive, with many users reporting increased job satisfaction and reduced frustration. Generative AI is still in its infancy but is becoming an integral part of business life.
Hype and Reality
Despite the excitement, deploying generative AI in business has proven more challenging than anticipated. Significant security, ethical, and practical concerns remain, including academic integrity issues, copyright disputes, and the reliability of AI-generated content. Large language models (LLMs) can produce “hallucinations” — confidently presented but incorrect information — which necessitates human oversight.
3 key Security Issues in Generative AI
- Data Leakage
Training data might contain personal or sensitive information that can surface during AI interactions. When models reproduce private information such as intellectual property or personal identities, it leads to confidentiality breaches. For example, last year, ChatGPT was tricked into generating Windows 10 and Windows 11 keys, leading to a significant security issues (Hackread). This can clearly damage trust and credibility and expose organisations to legal liabilities, regulatory fines, and reputational harm. - Prompt Sensitivity
User prompts can reveal sensitive business data, which may be processed and stored without encryption. According to a study by Menlo Security, 50.1% of employees surveyed were inputting personally identifiable information (PII), 24.6% were inputting confidential documents, and 5.3% were inputting payment card information (PCI). Leading to similar damaging outcomes as with data leakages – eroding trust, credibility, and exposing organistions to reputational harm. - Powerful Tool for Cybercrime
Generative AI can produce convincing content, such as phishing emails or sophisticated malware, that tricks users into disclosing sensitive information or evades traditional security measures. - In sensitive sectors like healthcare and finance, these security concerns limit the deployment of generative AI.
Building a secure future
There are many ways to combat security risks with generative AI, including educational awareness for employees, implementing robust data governance policies, and conducting regular security audits. Another key measure is investing in privacy-enhancing technologies. Let’s explore how some of these can address security concerns.
Privacy-Enhancing Technologies in Generative AI
Fully Homomorphic Encryption (FHE): Allows computations on encrypted data without decrypting it. This ensures that data remains confidential throughout AI processing, preventing exposure even during complex computations. When deployed in a scalable way, FHE can be used to submit encrypted prompts, and receive encrypted answers, thus protecting sensitive information passing through an LLM.
Federated Learning: Trains AI models on decentralised data sources without transferring sensitive data to a central location. This better protects data in Generative AI by keeping personal information on local devices, reducing the risk of data breaches.
Data Loss Prevention (DLP): Monitors and controls the movement of sensitive information. This helps prevent unauthorised access or leaks, ensuring that sensitive data is not inadvertently shared or lost.
Access Controls: Implements role-based or attribute-based permissions to ensure only authorised individuals can interact with sensitive data. This restricts access in generative AI applications, ensuring that only qualified users can handle sensitive information, thereby enhancing data security.
It’s likely that businesses will utilise a combination of measures, including privacy-enhancing technologies, to ensure security whilst using AI systems.
Final Thoughts
The critical privacy challenge in deploying generative AI today is ensuring the confidentiality of sensitive data while leveraging AI capabilities. This involves mitigating risks associated with data leakage, prompt sensitivity, and information retention within AI models.
To tackle these issues, we need a multifaceted approach including using privacy-enhancing technologies. Tools like FHE, federated learning, data loss prevention and access controls can help keep sensitive information safe. By focusing on these technologies, businesses can enjoy the benefits of generative AI whilst keeping valuable data secure and private. As these technologies evolve, they will play a crucial role in making generative AI both powerful and secure.